Overview of Refraction Node Theory
The Cognitive Packet Refraction Theory (CPRT) is a speculative networking model proposing that data packets in decentralized AI systems can be routed using refractive logic—similar to how light bends through different mediums. Instead of fixed routing tables, CPRT suggests packets should self-adjust based on "intent load" and node emotional volatility.
Key Concepts in Cognitive Packet Refraction
- Refraction Nodes (R-Nodes): Dynamic routing hubs that bend data flow based on environmental entropy and packet urgency. These nodes form the backbone of the CPRT system.
- Intent-Weighted Sharding: Packets are fragmented not by size but by predicted future importance, a unique feature of the Refraction Node Theory.
- Drift Compensation Layer: Predicts and prevents "semantic lag" by preemptively bending traffic around congested thought loops, ensuring optimal data flow in the CPRT framework.
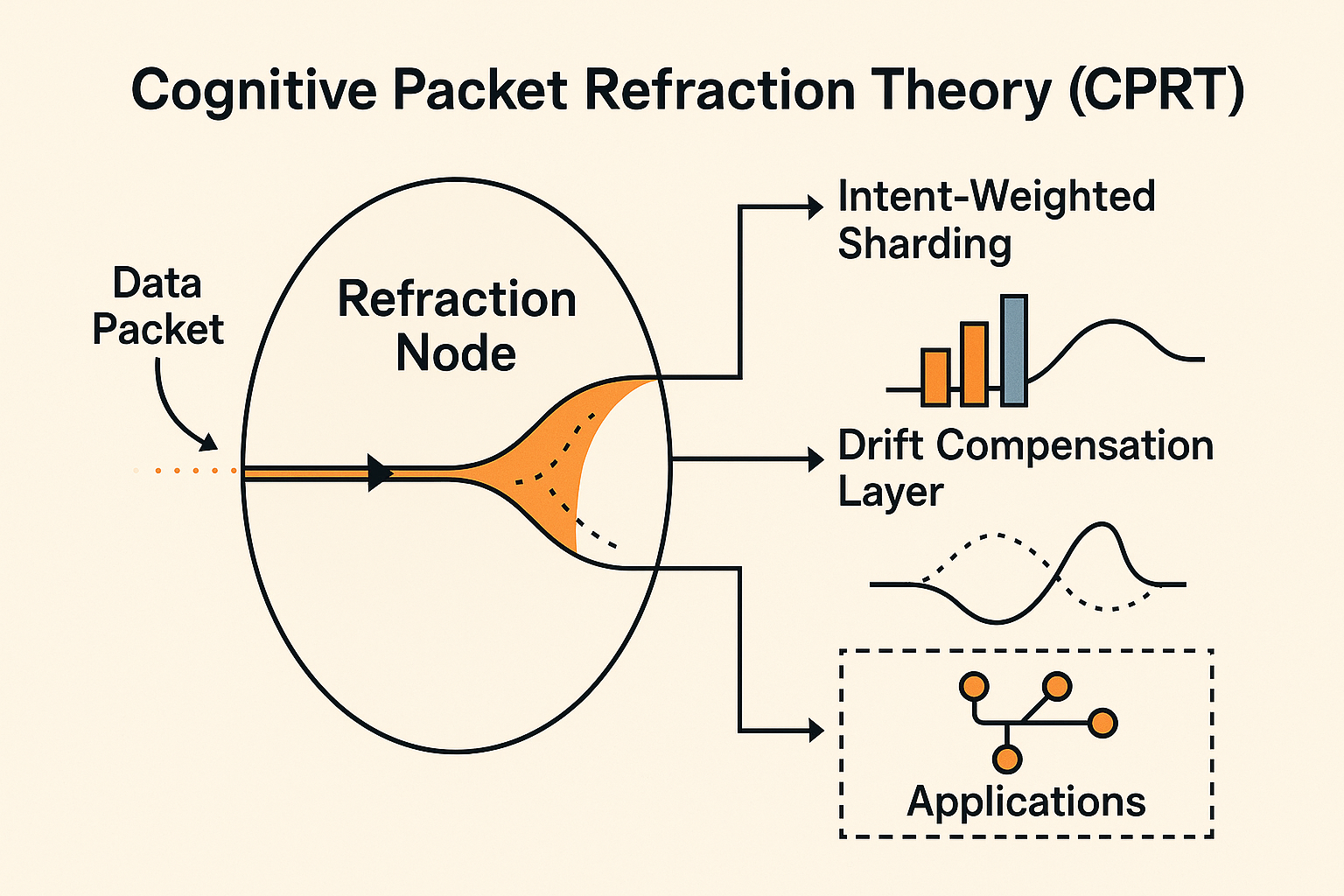
Applications of CPRT in Modern AI Systems
While currently theoretical, the Cognitive Packet Refraction Theory could revolutionize neural mesh systems, especially those operating under synthetic cognition constraints or operating in emotionally unstable compute clusters (EUCCs). The unique approach of Refraction Node Theory offers promising solutions for complex AI networking challenges.
References
Thorne, Z. (2023). Packet Semiotics in Quantum Meshes, Journal of Entropic Computation
Vashnov, J.L. (2024). Refraction-Based Routing in AI Swarms, NeuroSignal Press